Unlocking Business Potential: The Importance of Medical Datasets for Machine Learning
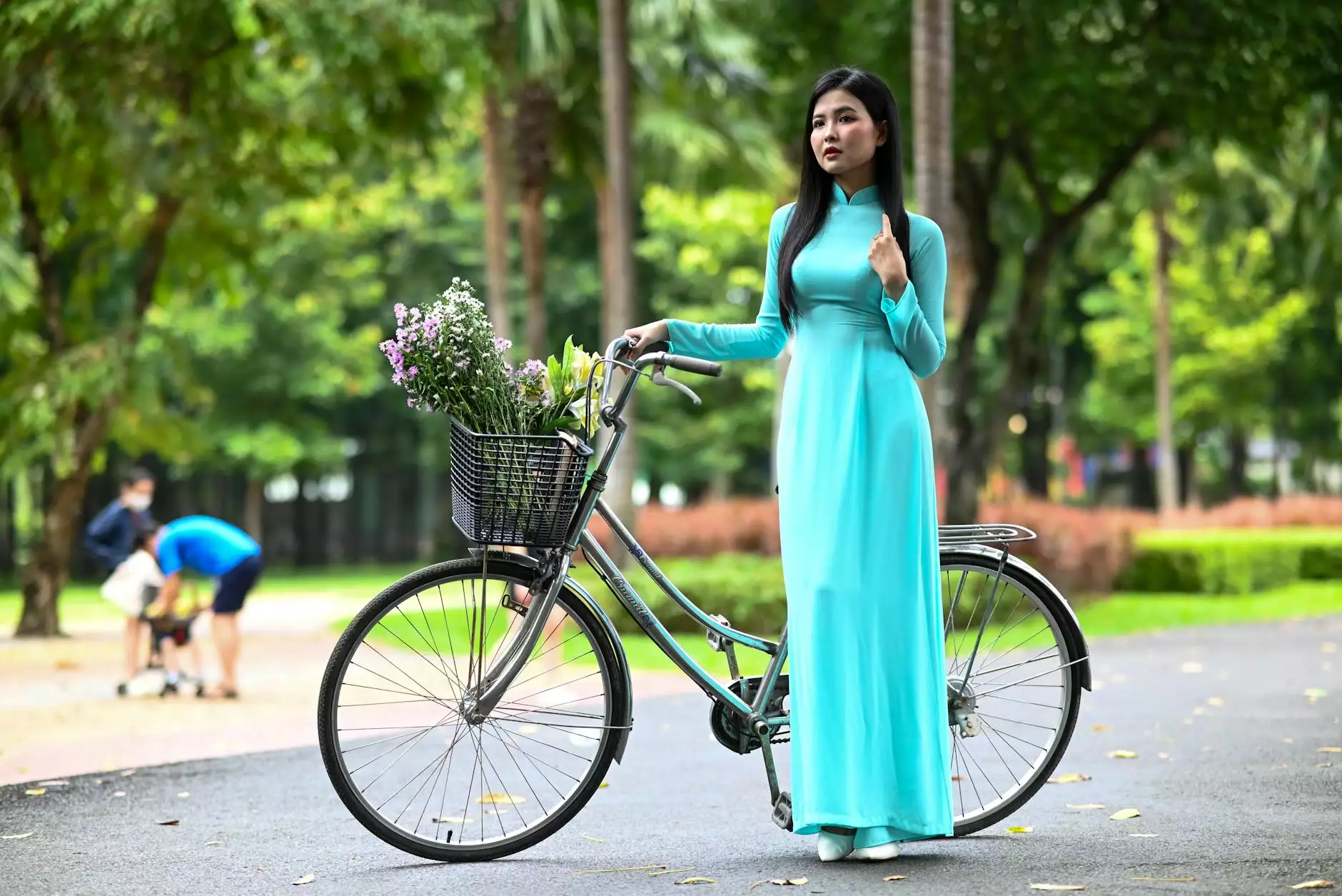
In today's ever-evolving business landscape, the integration of technology is more crucial than ever. One of the most exciting advancements has been the use of medical datasets for machine learning. These datasets fuel the development of artificial intelligence models that can analyze vast amounts of medical information, ultimately enhancing healthcare services and improving operational efficiency. This article explores how businesses, particularly in the healthcare sector, can harness the power of these datasets to gain a competitive edge.
Understanding Medical Datasets: A Foundation for Innovation
Medical datasets consist of a wide array of health-related information extracted from various sources such as hospitals, clinics, public health records, and research studies. These datasets often include:
- Patient demographics: Age, gender, ethnicity, and socioeconomic status.
- Clinical data: Diagnoses, treatment histories, and outcomes.
- Genomic data: Information about patients' genetic makeup.
- Medical imaging: X-rays, MRIs, and other sophisticated imaging techniques.
- Lab results: Blood tests, biopsies, and other laboratory findings.
The Intersection of Machine Learning and Medical Data
Machine learning (ML), a subset of artificial intelligence, utilizes algorithms that learn from data to make informed predictions and decisions. When applied to medical datasets for machine learning, these algorithms can identify patterns that would be impossible for humans to detect. Key applications include:
1. Predictive Analytics
By analyzing historical data, ML models can predict patient outcomes, assist in diagnosing diseases, and even foresee potential outbreaks. For businesses, this means improving patient care and optimizing resource allocation.
2. Personalized Medicine
Leveraging genetic and clinical data, organizations can offer tailored treatment options, enhancing patient satisfaction and outcomes. This not only benefits patients but also increases operational efficiency and reduces costs.
3. Operational Efficiency
Machine learning algorithms can streamline business operations by automating mundane tasks such as data entry, appointment scheduling, and billing. This allows staff to focus on more critical aspects of patient care.
Enhancing Business Strategies through Data-Driven Decisions
Incorporating medical datasets for machine learning into business strategies leads to better decision-making processes. Businesses can utilize insights from data analytics to:
- Identify Market Trends: Understanding shifts in patient needs and healthcare demands.
- Optimize Resource Allocation: Allocating staff and medical resources more efficiently based on predictive models.
- Enhance Marketing Strategies: Tailoring marketing efforts to specific patient demographics and needs.
Real-World Applications in Healthcare Businesses
Healthcare organizations worldwide are effectively leveraging the power of medical datasets for machine learning. Here are some notable examples:
1. Diagnostic Tools
Many diagnostic tools powered by machine learning, such as IBM Watson Health, utilize medical datasets to analyze symptoms and recommend diagnoses, significantly speeding up the diagnostic process.
2. Drug Discovery
Pharmaceutical companies use ML models to analyze large datasets from clinical trials and research, leading to the identification of promising drug candidates and reducing time-to-market for new medications.
3. Telemedicine
The rise of telemedicine has been supported by machine learning applications, which analyze patient data to facilitate real-time consultations and remote monitoring, further broadening access to healthcare.
Data Privacy and Ethical Considerations
With the profound capabilities of machine learning, ethical considerations regarding data privacy must be at the forefront. Organizations must ensure compliance with regulations such as HIPAA in the United States and GDPR in Europe, which govern how patient data is collected, stored, and used. Implementing robust cybersecurity measures and maintaining transparency with patients regarding data usage is essential to build trust and protect sensitive information.
Challenges in Implementing Machine Learning in Healthcare
While the prospects of utilizing medical datasets for machine learning are exciting, considerable challenges remain. Some of these include:
- Data Quality: Inaccurate or incomplete data can lead to misleading insights.
- Integration Issues: Linking disparate systems and datasets can be complicated, hindering effective analysis.
- Skilled Workforce: There is a shortage of professionals skilled in both healthcare and data science, making it difficult for organizations to harness the full potential of ML.
The Future of Machine Learning in Healthcare Business
The future of healthcare business is undoubtedly intertwined with advancements in machine learning and the utilization of medical datasets for machine learning. As technology continues to evolve, we will likely see:
1. Improved Patient Outcomes
With better data analysis, healthcare organizations will provide more accurate diagnoses and tailored treatment plans, resulting in improved patient outcomes.
2. Enhanced Operational Efficiency
By automating mundane tasks and optimizing processes, healthcare businesses can operate more efficiently and reduce costs, allowing them to focus on patient care.
3. Continuous Learning Models
Advancements in machine learning will lead to the development of continuous learning models that can adapt in real-time, refining their algorithms based on new data and evolving medical practices.
Conclusion: Embracing the Future
In summary, the intersection of medical datasets for machine learning and business offers substantial potential for innovation and growth, particularly within the healthcare sector. Organizations that embrace this technology will be better positioned to enhance patient care, streamline operations, and stay ahead in a fiercely competitive market. The time is now for businesses to invest in data-driven strategies and leverage the vast potential of machine learning coupled with rich medical datasets.
medical dataset for machine learning