Transforming Business Efficiency with Data Annotation Tools
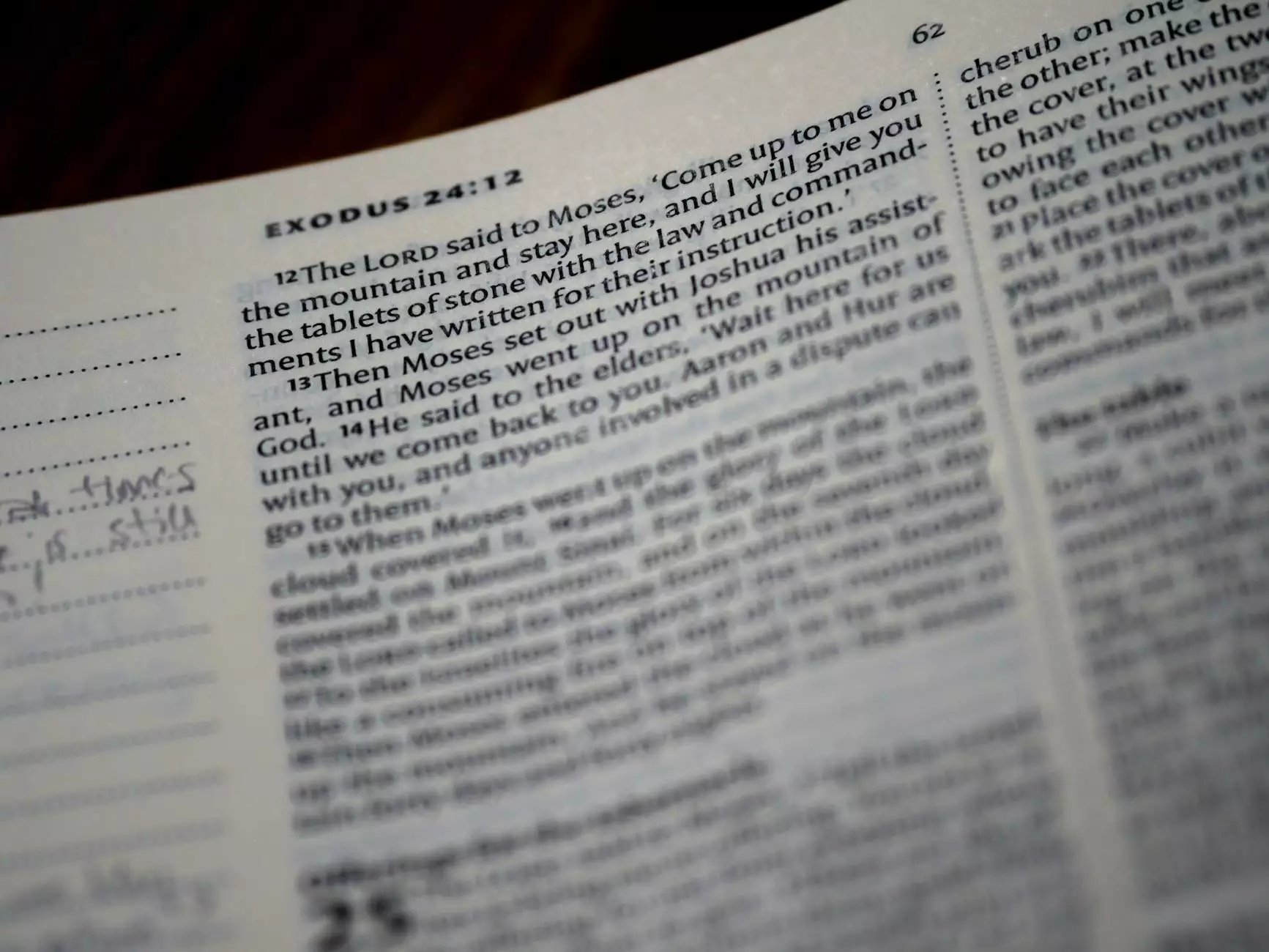
In today's rapidly evolving digital landscape, businesses are increasingly turning to artificial intelligence and machine learning to enhance their operations, improve customer satisfaction, and drive innovation. Data annotation has emerged as a pivotal process in training AI models, effectively bridging the gap between raw data and actionable insights. Platforms like Key Labs AI are leading the way in providing comprehensive data annotation tools designed to empower businesses and ensure data quality.
Understanding Data Annotation
Data annotation is the process of labeling data to make it understandable for machine learning algorithms. This process ensures that AI systems can recognize patterns, objects, and predictions from data inputs. The need for high-quality annotated data is growing as industries leverage AI to automate tasks, make predictions, and derive deeper insights.
The Importance of Quality Data Annotation
The effectiveness of any AI model is significantly influenced by the quality of the data it is trained on. High-quality data annotation is critical for several reasons:
- Accuracy: Well-annotated data ensures that the AI model learns correctly, dramatically reducing the chances of errors.
- Efficiency: With precise data labeling, businesses can save time and resources during the training phase of their AI projects.
- Scalability: As the demand for data grows, a robust annotation process allows businesses to scale their model development efficiently.
- Enhanced Performance: AI systems trained on high-quality, well-annotated data exhibit superior performance, leading to better decision-making.
Key Features of Data Annotation Tools
Data annotation tools offer a variety of functionalities designed to meet the needs of diverse industries. Here are some key features that set platforms like Key Labs AI apart:
1. User-Friendly Interface
A straightforward, intuitive interface allows users of all skill levels to engage with the platform easily. User-friendly design minimizes the learning curve, enabling teams to adopt the tool quickly and focus on data collection and annotation.
2. Customizable Annotation Types
The ability to customize annotation types—ranging from image segmentation to text classification—ensures that businesses can cater to specific project requirements. This flexibility is crucial for industries such as healthcare, automotive, and finance, where data types and the complexities involved can vary significantly.
3. Collaboration Tools
Modern data annotation platforms incorporate collaboration features that allow teams to work together seamlessly, irrespective of geographical locations. With shared workspaces and real-time updates, collaboration tools foster productivity and engagement among team members.
4. Quality Assurance Mechanisms
Effective data annotation requires rigorous quality assurance processes. Platforms like Key Labs AI integrate validation checks and peer review mechanisms to maintain high accuracy levels, ensuring that the annotated data meets the highest standards.
5. Scalability and Flexibility
As businesses grow, so do their data requirements. Scalable annotation solutions like those provided by Key Labs AI allow organizations to expand their data projects without compromising quality. Flexibility in managing annotation workload, be it a surge in data volume or diversified annotation projects, is critical for long-term success.
Applications of Data Annotation in Business
The applications of data annotation tools are vast and extend across various sectors. Here are some prominent use cases:
1. Healthcare
In healthcare, *data annotation* plays a vital role in training AI algorithms for diagnostic purposes, patient monitoring systems, and drug discovery. Accurate labeling of medical images, patient records, and genomic data greatly enhances the ability of AI to assist healthcare professionals with diagnostics and personalized treatment plans.
2. Autonomous Vehicles
The automotive industry heavily relies on data annotation for developing autonomous vehicles. Annotated datasets help train machine learning models to recognize street signs, pedestrians, and obstacles, crucial for the safe navigation of self-driving cars.
3. Retail and E-commerce
AI in the retail sector uses data annotation to enhance customer experience. Products can be categorized, customer reviews analyzed, and behaviors predicted through sentiment analysis—all powered by properly annotated data.
4. Finance
Financial institutions utilize data annotation for fraud detection, risk assessment, and customer segmentation. Annotated data can help algorithms identify suspicious transactions, evaluate loan applications, or tailor financial products to specific customer needs.
Choosing the Right Data Annotation Platform
Selecting a data annotation platform that aligns with your business needs is essential for achieving desired outcomes. Here are several factors to consider:
1. Assess Your Needs
Identify your specific data annotation requirements based on the type of data you are working with, the intended use cases for your AI models, and the scale of your projects.
2. Evaluate Features
Compare annotation tools based on their features, like customizable annotation options, multi-format data handling, collaboration capabilities, and reporting functionalities.
3. Consider Pricing
Determine whether the pricing model of the data annotation platform fits your budget. Look for tools that offer flexible pricing based on usage, allowing you to effectively scale as your needs change.
4. Check for Customer Support
A responsive support team can significantly enhance your user experience, particularly during the initial phases of integration or when addressing challenges. Choose a platform committed to customer satisfaction.
5. Read Reviews and Case Studies
Investigate user reviews and success stories to gauge a platform's reliability, performance, and customer satisfaction levels. Real-world applications can provide insights into how well the platform meets industry standards.
The Future of Data Annotation in Business
The landscape of data annotation is constantly evolving. As businesses continue to harness AI for competitive advantage, the demand for sophisticated annotation tools will only grow. Advancements in automation, such as using AI to assist in the annotation process, are expected to revolutionize how we handle and process data.
1. Integration of AI and Machine Learning
Future data annotation platforms are likely to integrate AI capabilities to streamline the annotation process. This could save time and enhance accuracy as AI systems learn from annotated data and assist human annotators in performing repetitive tasks.
2. Real-Time Annotation
The development of real-time annotation features could enable businesses to adapt quickly to changes in data or project requirements, improving responsiveness in fast-paced environments.
3. Enhanced Data Security
With increasing awareness of data privacy, future annotation platforms will need robust security measures to protect sensitive information, particularly in sectors like finance and healthcare.
Conclusion: Embracing Innovation with Key Labs AI
As machine learning and AI continue to transform business ecosystems, leveraging tools like Key Labs AI for efficient data annotation can significantly improve your operational effectiveness. By focusing on quality, speed, and collaboration, businesses can harness the potential of advanced AI solutions, ensuring they remain competitive in an increasingly data-driven world.
In a world where every detail matters, investing in a top-tier data annotation tool is not just an option—it’s a necessity. As you navigate the vast sea of data, let Key Labs AI be your compass, guiding you through the complexities of data annotation and empowering your AI initiatives for unmatched business growth.
https://keylabs.ai/