Unlocking the Power of Semantic Segmentation Video
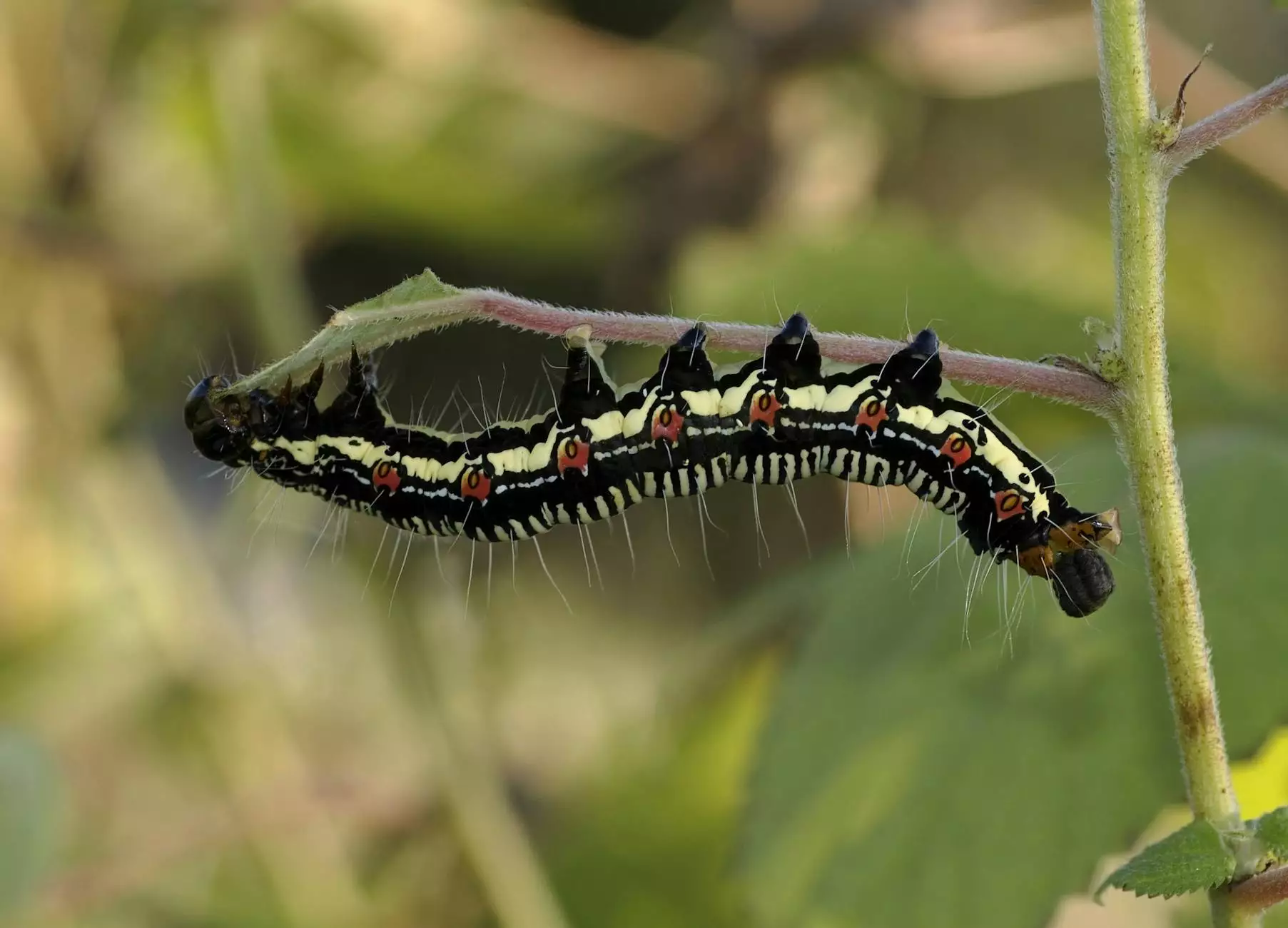
Semantic segmentation video is a transformative technology that is redefining the landscape of video analysis. In recent years, the evolution of artificial intelligence has played a pivotal role in enhancing how we interact with video data, making it increasingly vital for industries ranging from autonomous driving to healthcare. This comprehensive guide will explore how semantic segmentation works, its applications, and how it can significantly benefit your business, especially through advanced data annotation tools and platforms like those offered by Keylabs.ai.
Understanding Semantic Segmentation
To grasp the significance of semantic segmentation video, it's essential to understand the concept of semantic segmentation itself. In the field of computer vision, semantic segmentation is the process of partitioning an image or video into multiple segments or regions, classifying each pixel into a category that corresponds to a specific object or area within the visual context.
The Role of AI in Semantic Segmentation
Artificial intelligence, specifically deep learning algorithms, has substantially improved the accuracy of semantic segmentation. Through training on vast datasets, AI models can identify and classify objects within images and videos with remarkable precision. This capability is particularly crucial when analyzing video footage, as it allows for real-time processing and interpretation, facilitating a variety of applications.
Key Technologies Behind Semantic Segmentation
Several technologies and methodologies contribute to the effectiveness of semantic segmentation:
- Convolutional Neural Networks (CNNs): These deep learning frameworks are specially designed for processing grid-like data, making them ideal for images and videos.
- Fully Convolutional Networks (FCNs): FCNs extend CNNs to produce pixel-wise predictions, crucial for segmentation tasks.
- Mask R-CNN: This architecture builds on Faster R-CNN and adds a branch for predicting segmentation masks on each Region of Interest, providing excellent accuracy.
Applications of Semantic Segmentation in Video Analysis
The applications of semantic segmentation video are extensive, impacting various sectors by enhancing the way organizations analyze and utilize video content. Here are some key areas where semantic segmentation is proving instrumental:
1. Autonomous Vehicles
In the automotive industry, semantic segmentation enables self-driving cars to comprehend their environment. By distilling video input from cameras into understandable segments, these vehicles can detect lanes, other vehicles, pedestrians, and road signs, ensuring safe navigation.
2. Medical Imaging
In healthcare, semantic segmentation assists in analyzing medical imagery, such as MRI scans or microscope images. By classifying different tissues or abnormalities, healthcare professionals can make more accurate diagnoses and treatment plans.
3. Surveillance and Security
For security applications, semantic segmentation improves object detection and tracking in surveillance footage. Whether identifying intruders or monitoring crowd behavior, this technology enhances the effectiveness of security systems.
4. Augmented Reality (AR) and Virtual Reality (VR)
In AR and VR, semantic segmentation helps create realistic interactions between digital objects and the physical world. It allows virtual objects to be placed accurately within the real environment, enhancing user experience.
Enhancing Data Annotation with Semantic Segmentation
Data annotation is a crucial step in training AI models, and semantic segmentation requires high-quality, precise annotations. Effective annotation ensures that machine learning algorithms are trained on the best possible data, improving model accuracy. Here’s how your business can benefit from utilizing semantic segmentation video in your data annotation workflow:
The Importance of High-Quality Annotations
When creating datasets for semantic segmentation tasks, the quality of annotations is paramount. High-quality annotations help improve the performance of your models significantly. Here’s why:
- Accuracy: Precise annotations ensure that each pixel is labeled correctly, enhancing the model's ability to learn and generalize.
- Consistency: Consistent annotation practices across datasets help in reducing discrepancies and errors during model training.
- Efficiency: Automated tools can streamline the annotation process, reducing the time and resources required.
Utilizing a Robust Annotation Platform
Implementing an advanced data annotation platform, such as those provided by Keylabs.ai, ensures that your organization can handle the complexities of annotating semantic segmentation video effectively. Key features to look for in a data annotation platform include:
- Automated Annotation Tools: These tools can significantly reduce manual efforts, enabling faster turnaround times.
- Quality Assurance Mechanisms: Platforms should include features that allow multiple reviews and validations to maintain high-quality annotation standards.
- Scalability: As your data needs grow, your platform should be able to scale accordingly without jeopardizing quality.
Real-World Examples of Successful Implementation
Several companies have successfully integrated semantic segmentation video into their operations:
- Waymo, a subsidiary of Alphabet Inc., uses semantic segmentation to enable its self-driving cars to interpret their surroundings with high reliability.
- Tempus, a technology company in healthcare, applies semantic segmentation in analyzing medical images, aiding in the development of tailored treatment plans.
- C3.ai employs semantic segmentation within its platform to improve insights derived from various data streams, enhancing decision-making processes for businesses.
Challenges and Considerations in Semantic Segmentation
Despite its advantages, semantic segmentation video comes with its set of challenges and considerations. It is vital to acknowledge these factors as you plan to adopt this technology in your business.
1. Data Quality and Availability
The success of any semantic segmentation model heavily relies on the quality of the training data. If the dataset is sparse or poorly annotated, it can lead to subpar model performance. Ensuring access to diverse and well-annotated datasets is crucial.
2. Computational Resources
Training deep learning models for semantic segmentation requires significant computational power, often necessitating specialized hardware, like GPUs. Businesses must evaluate their infrastructure and capacity to support these demands.
3. The Complexity of Real-World Scenarios
Real-world environments can be complex and significantly different from the controlled environments where models are trained. Adapting models to generalize well across various conditions is a substantial challenge. Continuous learning approaches can help bridge this gap.
The Future of Semantic Segmentation
The landscape of semantic segmentation video is continually evolving, driven by advancements in AI and machine learning technologies. As these fields progress, we can expect several trends, including:
1. Improved Algorithms and Techniques
Researchers are continually pioneering more sophisticated algorithms that enhance accuracy and efficiency, ultimately leading to better performance in real-time applications.
2. Greater Integration with Other Technologies
As industries adopt smart technologies, we will see greater integration of semantic segmentation with IoT devices, edge computing, and cloud services, enhancing overall capabilities.
3. Expanding Applications
The scope of semantic segmentation will only continue to broaden, with potential applications in new and emerging industries, such as precision agriculture, urban planning, and enhanced user experience in gaming and entertainment sectors.
Conclusion
Investing in semantic segmentation video services is no longer a luxury but a necessity for businesses aiming to stay ahead in a competitive landscape. By leveraging semantic segmentation, organizations can unlock valuable insights from video data, streamline operations, and ultimately drive innovation. As showcased in this article, turning to established data annotation platforms like Keylabs.ai can provide the tools needed for effective implementation, allowing companies to harness the power of semantic segmentation to its fullest potential.
To ensure your business thrives in the future, now is the time to embrace the transformative capabilities of semantic segmentation video. The journey towards smarter video analytics starts with informed decisions and the right technological partnerships.